今回は TensorFlow.js の学習済みモデルの MobileNet を使用して画像分類を試してみます。 Angular で作っていますが、 Angular を使う必要はないです。
完成しているコードは下記にあります。
Angular-sample/tensorflow at main · tsuneken5/Angular-sample
Contribute to tsuneken5/Angular-sample development by creating an account on GitHub.
画像分類とは
画像分類とは、画像のパターンや特徴を捉えて、画像を特定のカテゴリに分類することです。
環境
- node: 18.20.0
- Angular CLI:17.0.0
- TensorFlow.js:4.22.0
- TensorFlow-model / MobileNet:2.1.1
GitHub - tensorflow/tfjs: A WebGL accelerated JavaScript library for training and deploying ML models.
A WebGL accelerated JavaScript library for training and deploying ML models. - tensorflow/tfjs
tfjs-models/mobilenet at master · tensorflow/tfjs-models
Pretrained models for TensorFlow.js. Contribute to tensorflow/tfjs-models development by creating an account on GitHub.
プロジェクトの作成
$ ng new tensorflow
パッケージのインストール
$ npm install @tensorflow/tfjs
$ npm install @tensorflow-models/mobilenet
/* To learn more about this file see: https://angular.io/config/tsconfig. */
{
"compileOnSave": false,
"compilerOptions": {
"outDir": "./dist/out-tsc",
"forceConsistentCasingInFileNames": true,
"strict": true,
"noImplicitOverride": true,
"noPropertyAccessFromIndexSignature": true,
"noImplicitReturns": true,
"noFallthroughCasesInSwitch": true,
"esModuleInterop": true,
"sourceMap": true,
"declaration": false,
"downlevelIteration": true,
"experimentalDecorators": true,
"moduleResolution": "node",
"importHelpers": true,
"target": "ES2022",
"module": "ES2022",
"useDefineForClassFields": false,
"skipLibCheck": true,
"lib": [
"ES2022",
"dom"
]
},
"angularCompilerOptions": {
"enableI18nLegacyMessageIdFormat": false,
"strictInjectionParameters": true,
"strictInputAccessModifiers": true,
"strictTemplates": true
}
}
tsconfig.json
に "skipLibCheck": true
を記載して MobileNet のコードでの型チェックをスキップさせます。
サービスの作成
判定に使う画像や結果を canvas を使用して表示するので、そのためのサービスを作成します。他にもローディングスピナーを呼び出すサービスなども作成してますが、そっちは本題ではないので省略します。
$ ng generate service service/canvas
public async uploadImage(imgStr: string, maxWidth: number, canvas: HTMLCanvasElement): Promise<void> {
const img = new Image();
const ctx = canvas.getContext('2d') as CanvasRenderingContext2D;
let scaleRaito: number = 1;
img.src = imgStr;
return new Promise((resolve, reject) => {
img.onload = () => {
let imageWidth = img.naturalWidth
if (imageWidth > maxWidth) {
imageWidth = maxWidth;
scaleRaito = imageWidth / img.naturalWidth;
}
canvas.width = imageWidth;
let imageHeight = img.naturalHeight * scaleRaito;
canvas.height = imageHeight;
resolve(ctx.drawImage(img, 0, 0, imageWidth, imageHeight));
};
img.onerror = (error) => {
console.log(error);
reject;
}
});
}
public drawLabel(label: string, x: number, y: number, canvas: HTMLCanvasElement): void {
const ctx = canvas.getContext('2d') as CanvasRenderingContext2D;
ctx.save();
ctx.font = '18px Meiryo';
ctx.textBaseline = 'top';
ctx.fillStyle = 'rgba(' + [0, 0, 255, 0.5] + ')';
let width = ctx.measureText(label).width;
ctx.fillRect(x, y, width, parseInt(ctx.font, 10));
ctx.fillStyle = '#fff';
ctx.fillText(label, x, y);
ctx.restore();
}
uploadImage
base64形式の画像を canvas に描画するためのメソッドです。
drawLabel
canvas に文字を描画するためのメソッドです。推定結果を出力するために使用します。
コンポーネントの作成
このコンポーネント以外にも画像アップロード用のコンポーネントやローディングスピナーなども作成してますが、そっちは本題ではないので省略します。
$ ng generate component component/page/mobilenet
import { Component } from '@angular/core';
import * as tf from '@tensorflow/tfjs';
import * as mobilenet from '@tensorflow-models/mobilenet';
import { ImageUploaderComponent } from '../../share/image-uploader/image-uploader.component';
import { LoadingSpinnerComponent } from '../../share/loading-spinner/loading-spinner.component';
import { LoadingSpinnerService } from '../../../service/loading-spinner.service';
import { CanvasService } from '../../../service/canvas.service';
@Component({
selector: 'app-mobilenet',
standalone: true,
imports: [ImageUploaderComponent, LoadingSpinnerComponent],
templateUrl: './mobilenet.component.html',
styleUrl: './mobilenet.component.css'
})
export class MobilenetComponent {
private model!: mobilenet.MobileNet;
private canvas!: HTMLCanvasElement;
constructor(
private loadingSpinnerService: LoadingSpinnerService,
private canvasService: CanvasService
) { }
private async loadModel(): Promise<void> {
this.loadingSpinnerService.show();
await tf.ready();
this.model = await mobilenet.load({ version: 2, alpha: 0.75 });
this.loadingSpinnerService.hide();
}
private async detect(): Promise<Array<{ className: string; probability: number; }>> {
const predictions = await this.model.classify(this.canvas);
return predictions;
}
public async startDetected(image: string): Promise<void> {
this.loadingSpinnerService.show();
const parent = this.canvas.parentElement as HTMLElement;
const width = parent.clientWidth;
await this.canvasService.uploadImage(image, width, this.canvas);
const result = await this.detect();
await this.canvasService.drawLabel(result[0].className, 0, 0, this.canvas);
this.loadingSpinnerService.hide();
}
async ngOnInit() {
this.loadModel();
}
ngAfterViewInit() {
this.canvas = document.getElementById('myCanvas') as HTMLCanvasElement;
}
}
loadModel
モデルをロードします。
version
・・・ ダウンロードするモデルを選択します。1で MobileNet v1 、2でMobileNet v2 を選択します。alpha
・・・ 0.25 (v1のみ), 0.5, 0.75, 1.0 から選択します。この値が大きいほど精度は上がります。
detect
canvas に描画した画像を MobileNet を使用して画像分類を行います。下記のような配列で結果が返ってきます。
[
{
"className": "Pembroke, Pembroke Welsh corgi",
"probability": 0.6058359146118164
},
{
"className": "Cardigan, Cardigan Welsh corgi",
"probability": 0.30394336581230164
},
{
"className": "red fox, Vulpes vulpes",
"probability": 0.0040221670642495155
}
]
実行結果
元画像
https://pixabay.com/photos/dog-corgi-cute-animal-4988985/
実行結果
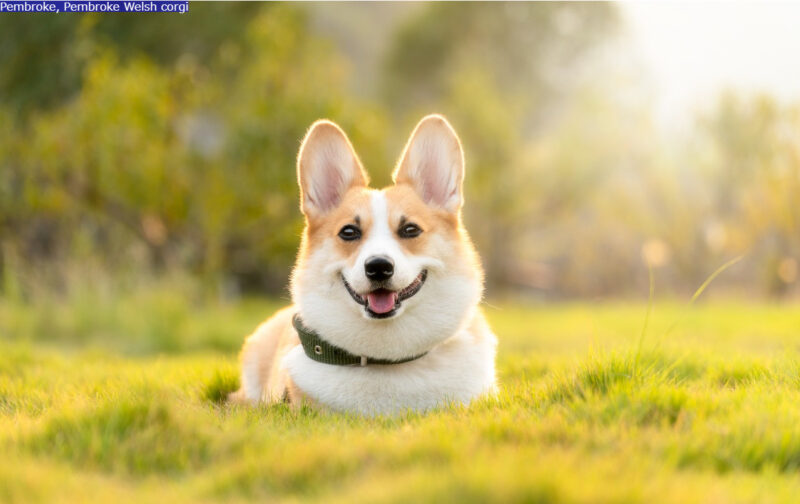
ImageNet は分類が細かすぎて出力結果があっているかどうかわかりにくい。。。
コメント